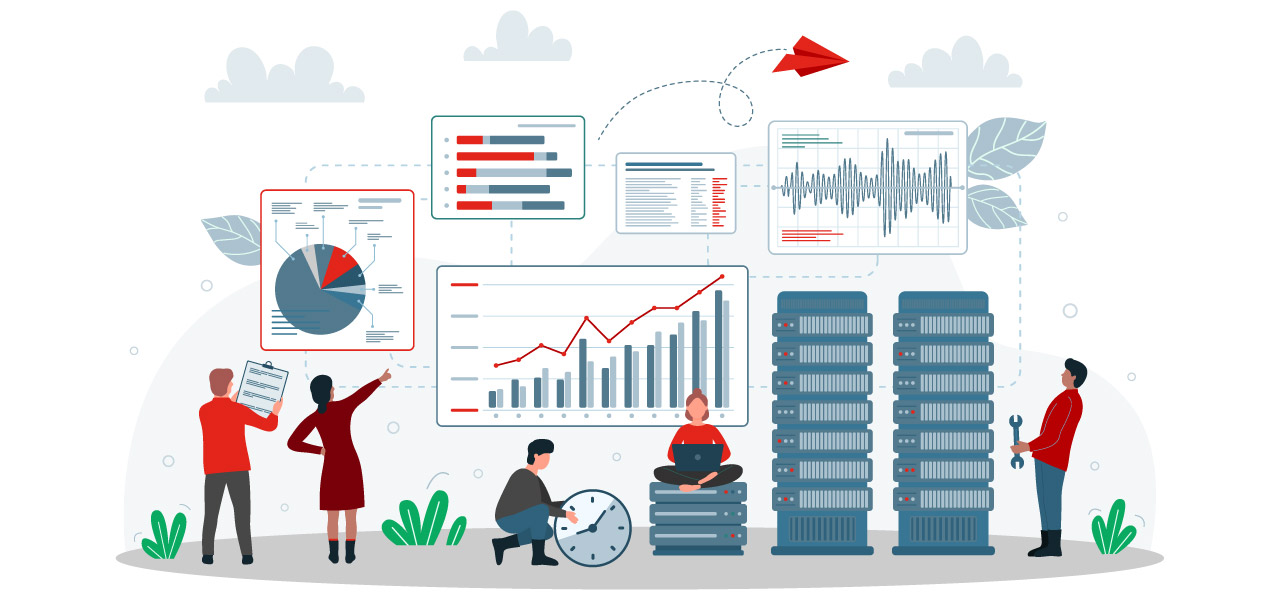
Historically, companies had two approaches to deriving value from data.
Some companies used a grassroots approach, where individual people and teams mined their own data stores for insights as needed. Other companies—usually larger ones—followed more of a “big-bang method,” in which the company formed a dedicated team to aggregate, prepare, and share data.
The problems with those approaches? Neither is sustainable. Neither can handle the amount of data coming in from customers and internal operations. And neither allows customers to benefit from their own data.
But now there’s a third option, according to McKinsey: establishing data as an internal product.
Using data as an internal product is fueling a competitive advantage for companies like Netflix, Intuit, PayPal, and many others.
Should you follow suit? A diverse group of industry experts answered that question in a recent roundtable conversation.
Panelists Sejal Amin, Chief Technology Officer at Shutterstock; Jana Eggers, CEO of Nara Logics; Razat Gaurav, CEO of Planview; and Dr. Rich Sonnenblick, Chief Data Scientist at Planview, were joined by moderator Ray Wang, Principal Analyst and Founder of Constellation Research, Inc.
This blog post delivers their perspectives on using data as a product, including:
- The shift in how companies view customer data
- How productizing data benefits companies and customers alike
- The importance of ethically sourcing data and gray areas to watch for
Listen to the full discussion: AI in the Enterprise: Opportunities, Challenges, and the Road Ahead
A shift in culture—and in mindset—on customer data
Moving data from on-premises to the cloud had myriad advantages for customers: easier data accessibility, better security, and more organization. The change also brought a massive shift in the way companies view, use, and manage data.
At the beginning of the cloud craze, companies like Planview began to store customers’ data. Many cloud companies approached customer data as a storage unit: you keep it, but you don’t touch it.
When companies were in a hands-off data mode, the developers, product teams, and customer support teams became used to never interacting with data without permission. Any access to data required explicit permission for specific actions.
But the culture has shifted. The relationship between companies and customers, as well as the relationship between teams within a company, has changed – primarily, because there’s more data available in real time.
Rather than being a storage unit service, cloud companies are now functioning more like banks – using data constantly to make better decisions for the company and for its customers.
Yes, companies store data, but they are also constantly using it, learning from it, and putting it to use. Treating data like a product means data gets its own processes, is customized for different custom uses, gets support (including documentation and interfaces) for different downstream needs, and is governed by clear rules – again, just like a bank.
Using data like a product benefits companies and customers alike
There’s value in harnessing data for product improvement, feature engineering, delivering AI/ML features to train models in real time, and making changes for downstream customer usage. Data is essential for analytics and optimization.
Despite these many benefits, using data as a product and viewing internal teams as customers are new mindsets for many companies. The larger the company, the bigger the lift required to create these data products in a systematic, repeatable, and efficient way.
As companies think of data more broadly, they can see opportunities to use data for more than internal use cases.
If a company is sitting on a pot of gold of customer data, it could be just the opportunity it needs to drive additional value from existing assets.
Customers are often doing their own data science and want access to their own data in an efficient way. Data as a product is yet another way companies can serve their customers, empowering customers to access their own data in more streamlined ways and improve their own processes.
It’s a win-win situation based on information that already exists and is just waiting to be used to its full potential.
For additional gains on the data front, companies are figuring out how to scale their offerings in more sustainable ways that require less human intervention and more automation and visibility to customers looking for different kinds of content.
It’s clear defining data as a product has internal benefits for a company. However, the same interpretation can also uncover a wide range of value for customers.
Ethical data sourcing couldn’t be more important
One prime example of using data as a product is Shutterstock. Sejal shared Shutterstock’s experience with ethically sourced data, as well as the concerns the industry has with treating data like a product.
Shutterstock engaged in several large deals with Amazon, Google, Meta, and many other large companies. Shutterstock sold them its library or subsets of its image library. In some cases, companies came back and asked for another type of asset or more of the same type of asset.
As companies consumed more data, Shutterstock was uniquely prepared with its ethically sourced assets and corresponding metadata, of which it already had a massive amount, both automatically and manually, through contributors.
It was exactly what hyper scalers needed to build their offerings. And, all of this was at a time when the conversation around both legal and ethically sourced data was heating up, so Shutterstock’s ethically sourced data was a competitive advantage.
There are some gray areas, though. When selling data like a product, Sejal encourages companies to ask themselves tough questions, such as:
- What are you giving away?
- How much of it are you giving away?
- Is this the right thing to be doing at this moment?
- What is the ultimate goal of what you are trying to do, and how are you trying to do it?
- What kind of content are you looking for? What kind of metadata are you looking for?
Digging into those answers will support companies in keeping ethical data sourcing as their north star.
Bringing it all Together
So, should you view data as a product? It’s a timely question, and the boom in AI capabilities accelerates the need to answer it.
The approach offers significant opportunities not only for your business but also for your customers. Ethical data sourcing supports a relationship with data that customers and stakeholders alike can trust and value.
Learn about the other implications of AI in today’s enterprise — including the impact on knowledge workers, responsible AI, and what organizations can do today — in AI in the Enterprise: Opportunities, Challenges, and the Road Ahead.