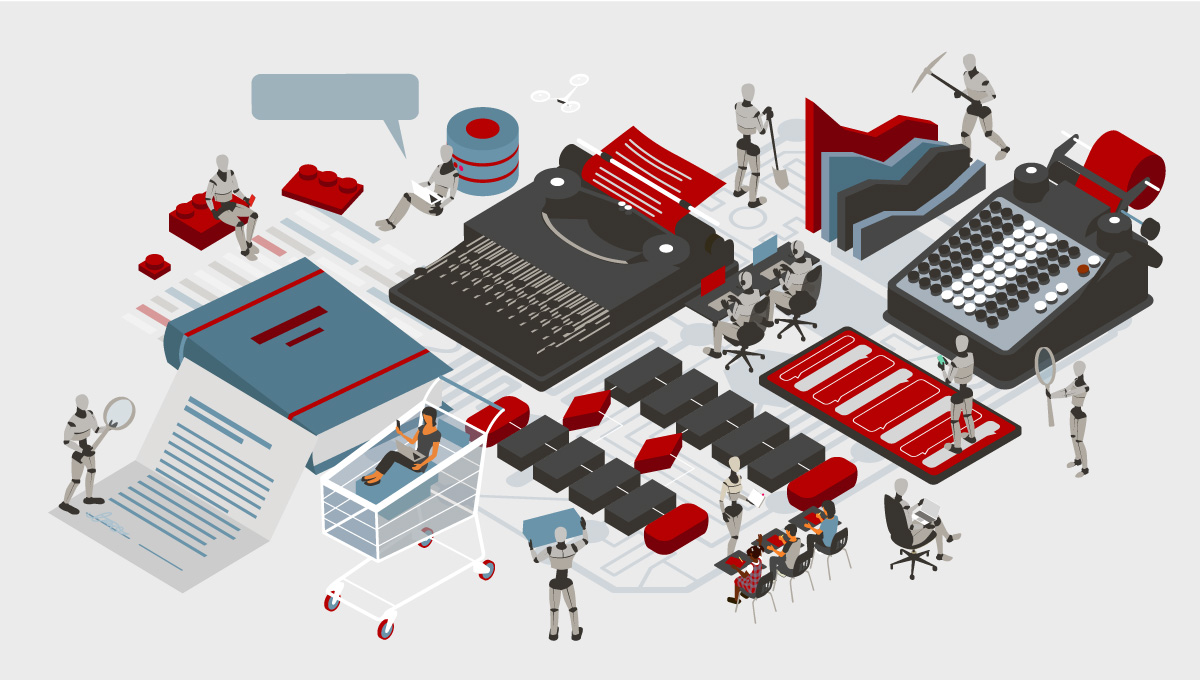
By now, many boards have asked their executive teams to create at least one AI use case—if not tens of use cases. Choosing the right AI use case doesn’t just affect how the board sees your performance, it impacts your enterprise’s competitiveness in the Digital Age.
It’s a remit that Jeff McMillan, the new head of firmwide AI at Morgan Stanley, understands. “We’ve deployed two use cases. But what about a world where we might have 100,000?” he said, in a recent interview with Axios.
The possibilities feel limitless; however, organizations can’t afford to be aimless. Aligning your AI use cases to your strategic goals and implementing them with a full picture of your risk exposure requires a careful decision-making process.
Whether you’re just entering the AI arena or it’s your umpteenth time returning, you’ll need a framework in place to gather ideas and prioritize the best ones, minimize the risk you’ll face, and continually assess your opportunities.
These executive insights from a recent panel discussion on AI in the enterprise provide the basis for an AI use case decision-making framework that can be applied to quickly identify the right use case.
How to Identify AI Use Cases
1. Crowdsource ideas.
To accelerate your decision-making process, start with a full basket of ideas. The best way to get them? Crowdsourcing.
Razat Gaurav, CEO of Planview, described how Planview surfaced AI use cases: “We leveraged one of our own tools called IdeaPlace to solicit all kinds of internal and external use cases from across our employee base.” Then, he went through a framework based on value to the company and complexity of effort to prioritize the results from the AI “hackathon.”
Jana Eggers has seen many AI implementations as CEO of Nara Logics, a software company that helps enterprises build AI advisors.
She’s noticed that when leaders excel with any AI product, they “go out and ask for all the ideas, and then they weigh those ideas against each other.” When she sees them struggle, one factor is that “they [didn’t] ask a broad enough perspective. . . gather those ideas from a larger group and weigh internal and external [use cases] together.”
You may not need this step each time you go through the use case framework if you still have a variety of fresh ideas from diverse sources.
2. Prioritize by pain point.
The next (crucial) step in the framework: prioritize by pain point, so that you’re orienting around business problems, rather than FOMO. Obviously, you don’t want to head down a use case path where the level of effort preponderates the pain you’re solving.
Be very intentional about choosing use cases connected to pain points.
Dr. Rich Sonnenblick, Chief Data Scientist at Planview, warned against choosing solutions merely because they have AI in their title or seem on trend right now. Instead, he urges executives to “be very intentional about what use cases you feel are connected to pain points within your organization and which use cases for your customers are solidly connected to their pain points.”
Ray Wang, moderator of the panel and Principal Analyst, Founder, and Chairman of Constellation Research, presented a hierarchy for aligning your use cases to different needs of the organization. Find his hierarchy and more insights in the full panel discussion on AI in the Enterprise.
3. Consider the ongoing cost.
As you decide where to invest in AI, include the ongoing cost of maintenance in your plans. If you decide to build it yourself, you face the initial cost of enabling the solution. Rich laid out the hidden ongoing costs:
- The cost of the support and maintenance over time that it takes to train the AI model continually.
- The time it takes to keep abreast of new model improvements that need to be folded in.
If you purchase a solution, it’s easier to spot the ongoing costs that will factor into your decision. After all, the money you spend maintaining one AI use case is less than you would have to spend on a new AI investment.
4. Start small and get feedback.
You can undertake an extensive, expensive market research exercise for AI use cases that takes eight to 10 weeks. Or you can start building with the user insights you already have to get a capability to market.
Sejal Amin, Shutterstock’s Chief Technology Officer, described their process: “We actually spun up [an AI project] in weeks, and we got an early release to market within four months. . . . We thought we understood the use case well enough to get it to a small audience and start getting some feedback. . . With that feedback, we’ll start iterating.”
While there are many ways to build new products and services, Sejal encourages other executives “to start building and testing and scrappy ways with the use case in mind. . . . If you have just enough to start building, go there; start getting feedback, and you build from there.”
5. Reassess quarterly.
Finally, return to the framework every quarter to refresh your plan. The AI vendor and technology landscape is changing fast. Don’t hesitate to replan if needs have evolved, if there’s a better way to solve a use case, or if you can bundle in an additional pain point.
Select AI Use Cases that Differentiate
The end game with these AI use cases is to identify the ones that become your strategic differentiators, early.
Getting to that point requires investment to test what works and what doesn’t, but if you can make those investment decisions smartly, you can come up with some valuable learnings, successful products, and hopefully, differentiating abilities.
Catch the full panel discussion, where you’ll hear the panel explore:
- How AI is improving decision-making and execution
- Responsible AI
- AI’s impact on knowledge workers
- What enterprise organizations can do now
- AI’s implications for enterprise data
Go further in developing your enterprise’s AI strategy with our guide, Building an AI Strategy for Digital Transformation Success, and an AI Executive Briefing.