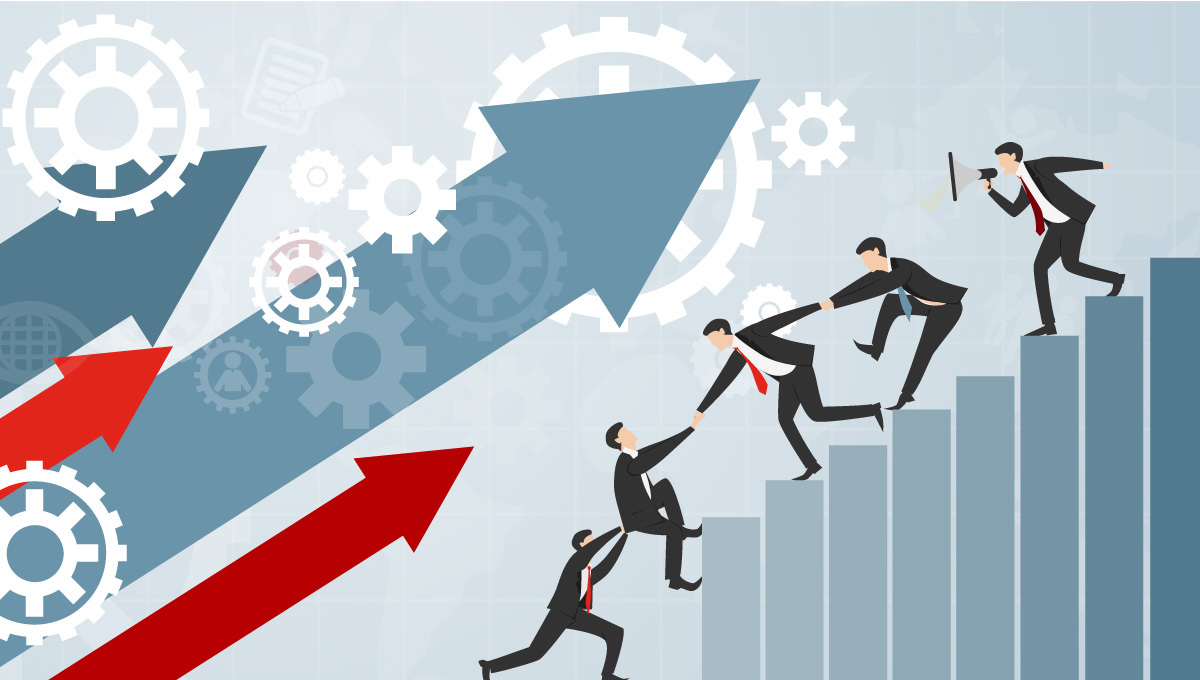
With a rapidly evolving digital landscape that continues to introduce waves of uncertainty, companies and organizations alike struggle for clarity and cognizance in organizational delivery for customer value.
It’s no surprise the abundance of moving parts contributes to an ever-ambiguous world for software delivery. With a multitude of products and services that companies serve to customers, the recognition of Value Stream Management (VSM) in modern software delivery has never been stronger.
To explain, VSM constitutes a set of management practices that focuses on maximizing the flow of business value within an organization with the end customer in mind. These constitute “value streams,” representing a team’s flow of connected work from customer request to product delivery. Any group suffering from the growing pains of scaling software delivery can attest to this value. But while the increasing number of companies adopting VSM has changed how teams build from project to product, a new innovative approach hits the spotlight: generative AI (genAI).
With the ability to analyze and interpret complex data, genAI has enabled both greater widespread VSM adoption and overall productivity and efficiency in the workplace. Before we go deeper, let’s establish what genAI is.
What is genAI?
GenAI has become a broad label, described as a type of artificial intelligence (AI) technology that can produce numerous types of content, such as text, video, image, and even music. In essence, AI models can take inputs in various forms and generate new content based on the modality of the model. For example, a large language model (LLM), a type of generative pre-trained transformer (GPT), is a form of text-based genAI that can output large bodies of creative text as if written by a human.
With text-based generation comes more multi-dimensional advanced technology that, as one can imagine, produces a multitude of content formats. With all the open-source code and available context across the internet, AI models can generate software code and help software developers debug their work.
Text-to-image AI technology, such as OpenAI’s DALL-E, leverages a multimodal implementation of AI models that can produce jaw-dropping images, art, and even realistic pictures that can easily be mistaken for the real thing. Text-to-video technology takes this up a notch, where video and image data undergo training to generate AI videos at medium-to-high fidelity.
This rapid advancement in genAI has disrupted many fields across both enterprise and consumer environments. It has not only impacted how we approach productivity and efficiency but also the ability to process valuable data for decision-making. AI models have proved their value rapidly, enabling productization. Organizations have begun to embrace this transformative technology in a way that can benefit everyone. Thus, one of the most profound examples lies in the world of VSM.
Benefits of GenAI in VSM
The foundation of VSM is about optimizing delivery for customer value. With every step of the way, the benefits of genAI fit like a glove.
First, you have the power of predicted analytics. Organizational and project data is essential to drive understanding of customer delivery and to act as a baseline for any team to understand their current and predicted outlook at any capacity. Incorporating genAI into analyzing organizational data to predict important trends, call out bottlenecks, and enable informed decision-making will revolutionize the pace of VSM execution and adoption.
For example, imagine you’re a manager of an engineering team migrating to a VSM platform like Planview Viz. With all the project data available to you (such as Jira work items, engineering capacity, and work completion time), genAI could instantly draw insights and analytical trends for faster decision-making, which leads to the second point.
Decision-making in VSM happens across all levels of an organization, from tactical solutions to higher-level strategy. Leaders of all engineering teams can benefit from more robust decision-making, thus extracting every ounce of value that VSM provides.
Whether a scrum master wants to identify and validate hidden bottlenecks in their team’s workflow or a director needs to analyze ROI data to decide whether their team needs to invest more capacity, genAI can save hours of time to do the analysis. Naturally, quicker decision-making positively influences everyone in a value stream through a cascading effect.
With the expectation that VSM can enable teams to identify their weakest points, genAI can elevate software development cycles by automating repetitive tasks and providing actionable suggestions on how a team can produce optimized customer value.
For example, imagine a team of engineers needs to do a manual review of their performance at the end of every sprint. What if an AI assistant, like Planview Copilot, could analyze their data and provide actionable suggestions to enhance their output for the next sprint?
3 Use Cases for GenAI in VSM
Of course, the above is only scratching the surface. A world of genAI in VSM will introduce more use cases to further enhance customer products and services. Below are some notable use cases that are predicted to play key roles in how VSM will shape the technological landscape.
- Optimizing resource allocation. Organizations have suffered for decades with suboptimal resource allocation. Foundationally speaking, VSM practices aim to uncover all information needed to make the right decision on resourcing and capacity; genAI, as one can imagine, makes this step significantly less cumbersome, both with identifying what data is needed to make the resourcing decision and analyzing the data itself.
- Automated documentation, reports, and release-notes generation. With a modern software development team comes modern practices on documentation, either for internal and/or external purposes. Likewise, reporting for managers and release notes for customers can be equally important for enterprise and consumer settings alike. Reducing the tedious, shallow work needed for these write-ups can be critical to improving both team performance and morale.
- Faster prototyping and experimentation. Especially in the case of teams needing to build new features or test ideas, genAI and its ability to output content in various forms has completely reformed the process. Whether it’s helping product and portfolio managers decide on the next solution to ideate or the generation of code to build concepts out, genAI will play a pivotal role in rapid prototyping and agile software development.
Challenges and Considerations
Perhaps the most critical piece of the AI adoption puzzle is data privacy, security, and ethics – subjects that continue to spark greater debate as AI-based products emerge.
GenAI, due to its complicated training process and implementation, is always causing concern regarding the handling of individual and company data. Leakage or recreation could damage intellectual secrets, especially at the enterprise level. The best approaches to privacy and security continue to evolve.
A blooming industry and community of best practices to minimize potential harm from the use of AI will greatly benefit the tech scene. Ethics, a separate but equally important topic, can entail unpredictable but potentially harmful effects at all organizational levels.
GenAI is founded on its creativity and expansive data, which can sometimes result in a double-edged sword. For instance, AI models could generate content that is offensive, abrasive, or unethical, leading to a wave of negative repercussions. This could harm the reputation of an organization or individual and also have adverse mental effects on anyone who encounters it.
Another challenge worth noting is the ongoing battle over models and pricing, which has fueled intense competition among AI producers in the market. It’s important for companies – especially those undergoing VSM transformations – to adopt AI products and/or models that fit specific use cases or needs the best. Various models in today’s AI market are trained on different data sets and have been tuned and tailored for various purposes.
Conclusion
Without a doubt, the rapid advancement of genAI, encompassing text, image, and video generation, has ushered in transformative changes across various sectors. In the accelerating world of VSM, AI introduces significant benefits, revolutionizing the execution and adoption of VSM practices that positively impact customer value.
The benefits of incorporating genAI into VSM are multifaceted. AI has already proven its endless capability, whether it features predicted analytics to drive greater decision-making at all organizational levels or the automation and suggestion of actionable steps to conquer a team’s biggest bottlenecks.
With more use cases like resource optimization and rapid experimentation on the horizon, both genAI and VSM will act as mainstays in the years to come. The convergence of both promises unparalleled advancements in efficiency, decision-making, and increased company output in quality and quantity.
To stay competitive in today’s dynamic market, organizations are encouraged to explore AI-driven VSM solutions, like Planview Copilot, to unlock the full potential of this transformative technology for enhanced productivity and strategic advantage. Watch the demo and join Planview’s Early Access program to learn more.